Multimodal language models have made remarkable strides in processing and generating content across various formats, but are they truly equipped to handle visual data effectively? While these models excel at textual analysis and generation, their visual capabilities often fall short of expectations. The phrase "eyes wide shut" aptly describes this paradox, where models seem to "see" but fail to interpret visual information accurately. As we explore the visual shortcomings of multimodal LLMs, it becomes clear that these systems require significant refinement to bridge the gap between textual and visual understanding. This article dives deep into the challenges, limitations, and potential solutions for enhancing the visual intelligence of these advanced models.
The rapid evolution of artificial intelligence has brought multimodal LLMs to the forefront of technological innovation. These models are designed to process multiple types of data, including text, images, and even audio. However, their performance in the visual domain remains a contentious issue. Despite their ability to generate descriptive captions or classify images, these models often struggle with nuanced visual contexts, leading to errors and misinterpretations. The implications of these limitations extend beyond technical performance, impacting applications in healthcare, autonomous systems, and content creation. Understanding the root causes of these shortcomings is crucial for advancing the field.
As researchers and developers grapple with the complexities of multimodal learning, the need for a comprehensive evaluation of visual capabilities becomes increasingly apparent. By examining the underlying architecture, training methodologies, and data sources, we can identify the factors contributing to the "eyes wide shut" phenomenon. This article aims to shed light on these issues, offering insights into the current state of multimodal LLMs and the pathways toward improvement. For businesses, researchers, and enthusiasts alike, understanding these limitations is essential for harnessing the full potential of these transformative technologies.
Read also:Hdhub4u In Movies Bollywood Your Ultimate Guide To Highquality Bollywood Entertainment
What Are the Key Challenges in Visual Processing for Multimodal LLMs?
Multimodal LLMs face several challenges when processing visual data, primarily due to the complexity of interpreting contextual information. Unlike textual data, which is inherently sequential and structured, visual data requires the model to recognize patterns, relationships, and spatial arrangements. For instance, a model may correctly identify an object in an image but fail to understand its role within the scene. This disconnect highlights the need for more sophisticated visual processing mechanisms. Additionally, the lack of diverse and high-quality training datasets exacerbates the problem, as models trained on limited data often exhibit biases and inaccuracies.
Why Does "Eyes Wide Shut" Persist in Multimodal Models?
The "eyes wide shut" phenomenon refers to the inability of multimodal LLMs to fully comprehend visual information despite their apparent ability to "see." This issue arises from several factors, including insufficient cross-modal alignment and inadequate attention mechanisms. Cross-modal alignment ensures that textual and visual data are processed in harmony, enabling the model to generate coherent outputs. However, many models struggle with this alignment, leading to inconsistencies in their outputs. Furthermore, attention mechanisms, which prioritize relevant parts of an image, often fail to capture the nuances of complex scenes. Addressing these challenges requires a combination of architectural improvements and innovative training strategies.
How Can We Enhance the Visual Capabilities of Multimodal LLMs?
Improving the visual capabilities of multimodal LLMs involves a multi-faceted approach. One promising direction is the development of hybrid architectures that combine the strengths of convolutional neural networks (CNNs) and transformers. CNNs excel at extracting spatial features from images, while transformers are adept at handling sequential data. By integrating these two paradigms, researchers can create models that better understand both visual and textual contexts. Additionally, expanding the diversity of training datasets and incorporating real-world scenarios can help mitigate biases and enhance performance. These advancements are critical for overcoming the "eyes wide shut" limitations of current models.
What Role Does Data Quality Play in Visual Processing?
Data quality is a cornerstone of effective visual processing in multimodal LLMs. High-quality, diverse datasets enable models to learn a wide range of visual patterns and contexts, improving their ability to generalize across different scenarios. Conversely, poor-quality or biased datasets can lead to subpar performance and reinforce existing limitations. Ensuring data quality involves rigorous curation, annotation, and validation processes. Researchers must also prioritize ethical considerations, such as avoiding harmful biases and ensuring inclusivity in dataset representation. By focusing on data quality, we can significantly enhance the visual capabilities of these models.
Exploring the Eyes Wide Shut? Eploring the Visual Shortcomings of Multimodal LLMs
As we delve deeper into the "eyes wide shut" phenomenon, it becomes evident that the challenges facing multimodal LLMs are multifaceted. Beyond technical limitations, there are broader implications for the applications and adoption of these models. For instance, in healthcare, accurate visual interpretation is crucial for diagnosing diseases from medical images. Similarly, in autonomous systems, reliable visual processing ensures safe navigation and decision-making. Addressing these challenges requires a collaborative effort from researchers, developers, and industry stakeholders. By fostering innovation and sharing knowledge, we can pave the way for more robust and capable multimodal models.
Why Are Multimodal Models Struggling with Complex Visual Contexts?
Complex visual contexts pose significant challenges for multimodal LLMs, as they require a deep understanding of spatial relationships, object interactions, and environmental cues. Many models struggle with these aspects due to their reliance on simplistic feature extraction techniques and insufficient contextual awareness. For example, a model may fail to recognize that a person is holding an umbrella in a rainy scene, leading to incorrect interpretations. Overcoming these limitations necessitates advancements in both model architecture and training methodologies. Techniques such as attention-based reasoning and contextual embedding can help bridge the gap between basic feature extraction and advanced visual understanding.
Read also:Tyler James Williams Father Unveiling The Life And Legacy Behind The Scenes
Can Multimodal LLMs Achieve True Visual Intelligence?
The quest for true visual intelligence in multimodal LLMs is a formidable challenge, but not an insurmountable one. Achieving this goal requires a fundamental shift in how we approach visual processing and model development. Researchers must focus on creating models that not only recognize objects and scenes but also understand their significance and relationships. This involves integrating advanced cognitive processes, such as reasoning and memory, into the model architecture. By doing so, we can move closer to realizing the full potential of multimodal LLMs and overcoming the "eyes wide shut" limitations that currently hinder their performance.
What Are the Practical Implications of Visual Limitations in Multimodal Models?
The visual limitations of multimodal LLMs have far-reaching practical implications across various industries. In content creation, for example, models with poor visual understanding may generate inaccurate or misleading captions, impacting user experience. In autonomous systems, inadequate visual processing can compromise safety and reliability, posing significant risks. Addressing these implications requires a proactive approach, involving continuous improvement of model capabilities and rigorous testing in real-world scenarios. By prioritizing these efforts, we can ensure that multimodal LLMs meet the demands of modern applications and deliver value to users.
Table of Contents
- Eyes Wide Shut? Delving into the Visual Limitations of Multimodal LLMs
- What Are the Key Challenges in Visual Processing for Multimodal LLMs?
- Why Does "Eyes Wide Shut" Persist in Multimodal Models?
- How Can We Enhance the Visual Capabilities of Multimodal LLMs?
- What Role Does Data Quality Play in Visual Processing?
- Exploring the Eyes Wide Shut? Eploring the Visual Shortcomings of Multimodal LLMs
- Why Are Multimodal Models Struggling with Complex Visual Contexts?
- Can Multimodal LLMs Achieve True Visual Intelligence?
- What Are the Practical Implications of Visual Limitations in Multimodal Models?
- Conclusion: The Path Forward for Multimodal LLMs
Conclusion: The Path Forward for Multimodal LLMs
The "eyes wide shut" phenomenon serves as a poignant reminder of the challenges that persist in the realm of multimodal LLMs. While these models have made impressive strides in processing textual and visual data, their limitations highlight the need for continued innovation and refinement. By addressing the key challenges in visual processing, enhancing data quality, and fostering collaboration among stakeholders, we can pave the way for more capable and reliable models. The future of multimodal LLMs lies in achieving true visual intelligence, enabling them to seamlessly integrate with human perception and understanding. As we embark on this journey, the potential applications and benefits of these models are boundless, promising to transform industries and enhance our daily lives.
As we conclude our exploration of the visual shortcomings of multimodal LLMs, it is important to recognize the progress that has been made and the opportunities that lie ahead. By embracing the "eyes wide shut? eploring the visual shortcomings of multimodal llms" paradigm, we can unlock new possibilities and drive the field forward. Whether through advancements in architecture, training methodologies, or data quality, the path forward is clear: a commitment to excellence, innovation, and collaboration. Together, we can ensure that multimodal LLMs not only "see" but truly understand the world around them.
In the grand scheme of artificial intelligence, the journey to overcome the "eyes wide shut" limitations of multimodal LLMs is just beginning. With each breakthrough and discovery, we inch closer to realizing the full potential of these transformative technologies. As researchers, developers, and users, we have a shared responsibility to push the boundaries of what is possible and create solutions that benefit society as a whole. The eyes of the future are wide open, and the possibilities are endless.
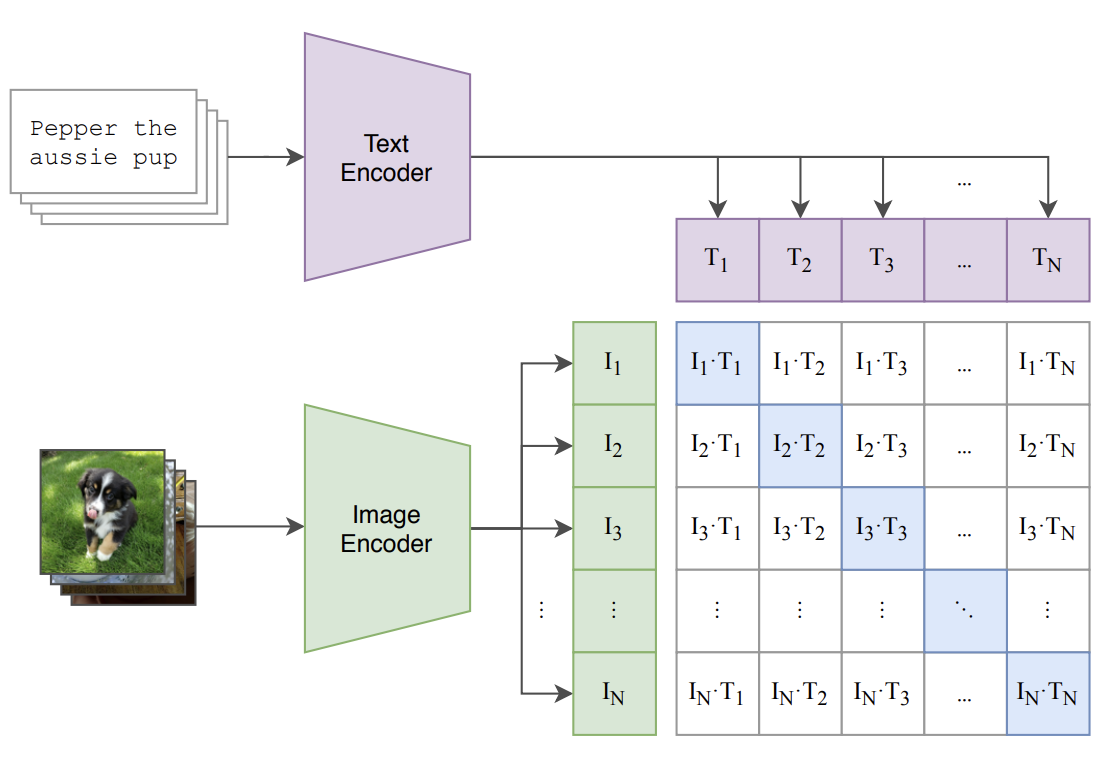